A distributed key-value store is a system that stores data in a key-value format across multiple nodes. It is a fundamental building block for distributed systems and is used in a variety of applications like caching, configuration management, and service discovery.
Single Node Key-Value Store vs Distributed Key-Value Store
A Single Node Key-Value Store is a simple system that stores key-value pairs in memory or on disk.
Limitations:
- Single point of failure
- Only vertical scaling is possible
A Distributed Key-Value Store overcomes these limitations by distributing data across multiple nodes.
Advantages:
- High availability and fault tolerance
- Horizontal scaling
- Load balancing
Challenges:
- When there are multiple nodes, how do we decide which node to store the data on?
- How do we ensure that the data is consistent across all nodes?
- How do we handle node failures?
- How do we handle network partitions?
- In any distributed system, we need to consider CAP theorem.
What is CAP theorem?
CAP theorem states that in a distributed system, we can only achieve two out of the following three guarantees:
- Consistency: All nodes see the same data at the same time.
- Availability: Every request gets a response, even if some nodes are down.
- Partition tolerance: The system continues to operate despite network partitions.
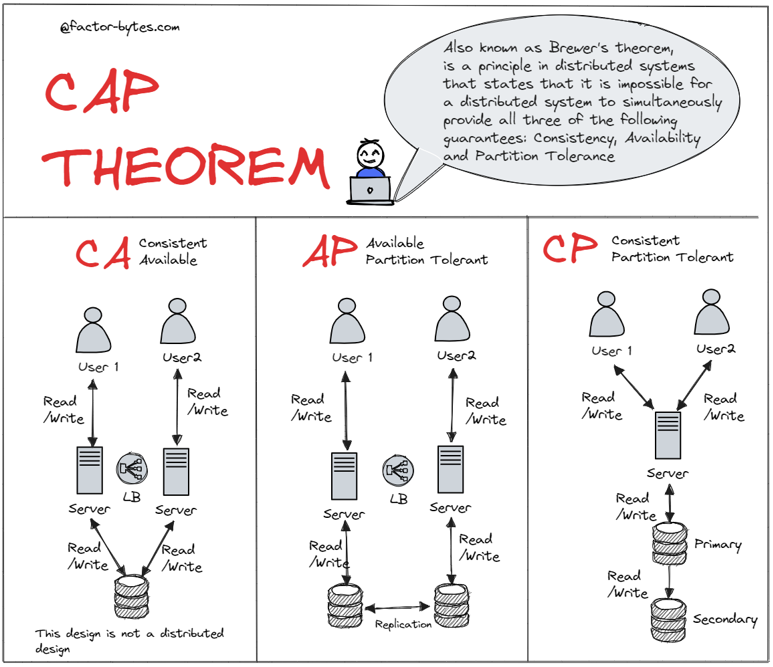
System Requirements for a Distributed KV Store
- Scalability: The system should be horizontally scalable, allowing the addition of new nodes to handle increased load.
- Consistency: Ensure strong consistency guarantees across all nodes in the system, even in the presence of failures and network partitions.
- Fault Tolerance: Implement mechanisms to handle node failures gracefully and maintain data availability and integrity.
- Concurrency: Support concurrent read and write operations efficiently while maintaining data consistency.
- Partitioning: Implement data partitioning strategies to distribute key-value pairs evenly across multiple nodes.
System Design
Components
- Client: The client sends read and write requests to the distributed key-value store.
- Load Balancer: Distributes incoming requests across multiple nodes to ensure even load distribution.
- Node: Each node in the distributed key-value store is responsible for storing a subset of the key-value pairs.
- Replication Manager: Handles replication of data across multiple nodes to ensure fault tolerance and data availability.
- Consistency Manager: Ensures strong consistency guarantees across all nodes in the system.
- Failure Detector: Detects node failures and triggers recovery mechanisms to maintain data availability and integrity.
- Partition Manager: Determines which node should store a given key-value pair based on a partitioning strategy.
info
Points 3, 4, 5, and 6 can be implemented using a Consensus Algorithm like Raft or Paxos.
Algorithm of my choice: Raft
Raft is a consensus algorithm that ensures strong consistency guarantees in a distributed system. It elects a leader among the nodes, which is responsible for coordinating read and write operations. Raft ensures that all nodes in the system see the same data at the same time, even in the presence of failures.
Short Overview of Raft
- Each node in the system can be in one of three states: Leader, Follower, or Candidate. There is only one leader at any given time.
- The Leader sends heartbeats to Followers to maintain its leadership status.
- If a Follower does not receive a heartbeat within a certain time frame, it becomes a Candidate and initiates an election.
- The Candidate requests votes from other nodes in the system to become the Leader.
- Once a Candidate receives a majority of votes, it becomes the Leader and coordinates read and write operations.
- Each Node stores a log of all write operations, and the Leader replicates this log to all Followers to ensure data consistency.
- When a new node joins the system, it requests the log from the Leader to catch up with the current state.
- When a failed node recovers, it requests the missing log from the Leader to synchronize its state with the rest of the system.
Datastructure used in the system
- Key-Value Store: A simple HashMap data structure to store key-value pairs.
- Log: A simple index-based array to store write operations in the system.
Live Demo - Scenario 1: Replication of Data
Live Demo - Scenario 2: Scaling the System
Live Demo - Scenario 3: Network Partitioning
Demo not available for this scenario.
Conclusion
In this blog post, we explored the design of a distributed key-value store using Raft as the consensus algorithm.